Toshiba's GridDB is an innovative, high-performance, highly scalable database that meets all the requirements of IoT (Internet of Things) systems a data model and processing capabilities optimized for time-series data, the scalability to handle explosively growing data volumes, and high availability to support stable operation. Responding to the deregulation of electrical power, an electric power company, operating a wheeling system, chose GridDB as the processing platform to handle data sent every thirty minutes from millions of smart meters. In this case, one of the pioneering systems of the IoT age was introduced; immediately processing the large amounts of time-series data received in frequent intervals. Let's look at this example of GridDB use case to ensure that GridDB's overwhelming performance could be leveraged in operation site and the importance of system integration coordination with existing technologies.
Smart meters, supporting full-fledged liberalization of electrical power retailing
Deregulation of electrical power began in March 2000. The first step was enabling large-scale factories, department stores, office buildings, and other customers using extra high voltages (20kV or more) to freely select their power companies. At the same time, they also became able to purchase power from new electrical power retailers. In 2004 electrical power deregulation was extended to small and medium-sized factories and buildings using high voltages (6kV or more). In April 2016 the process was completed by expanding policies to all electrical power users; including households and businesses using low voltages (100/200V) can select their power companies. This process was the full retail liberalization of electrical power.
Until then electrical power market was a monopoly, but this deregulation brought competition to the market. Power companies turn around their direction and start operating their businesses by focusing consumers benefit. They now had to compete in terms of prices and services with newcomers, such as communications companies, gas companies, and trading companies, from fields other than the electrical power business.
Smart meters, new power meters, were essential to integrate electrical power business that reflects consumers' needs. Power companies replaced conventional power meters in homes, stores, and the like, with smart meters to measure usage every 30 minutes and to send this data (meter data) to a concentrator. This made it possible not only to automate meter reading, simplify ampere change work through remote operation, and speed up recovery from power outages, but also to create new services such as assisting customers by offering power saving proposal through electricity usage visualization service. The deregulation of electrical power retailing made the new wheeling systems essential for power companies. For fair competition, the power companies are required to receive and rapidly process meter data and disclose information such as data tabulation results and power system usage fees (wheeling fees) to new entrants. Thus smart meters are considered platform system for fare competition (Fig. 1).
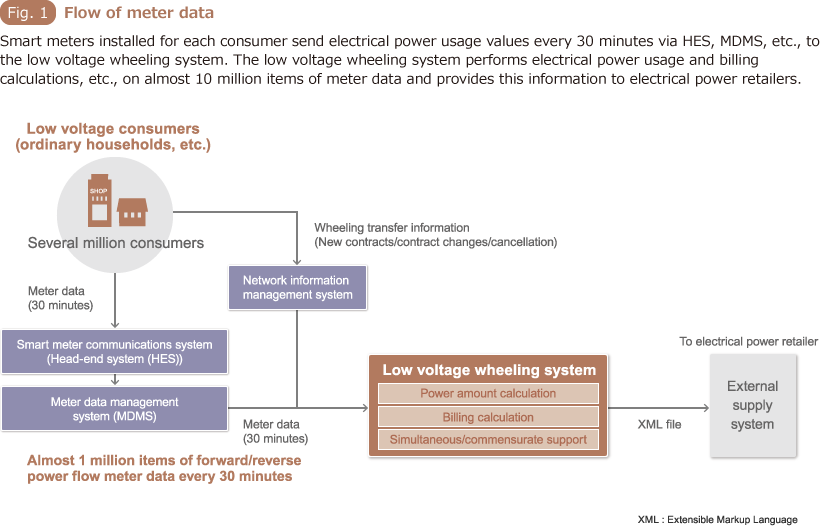
One power company was quick to respond to these dynamic changes.
Click here to move to the top of this page.
The big data processing issues uncovered by verification tests
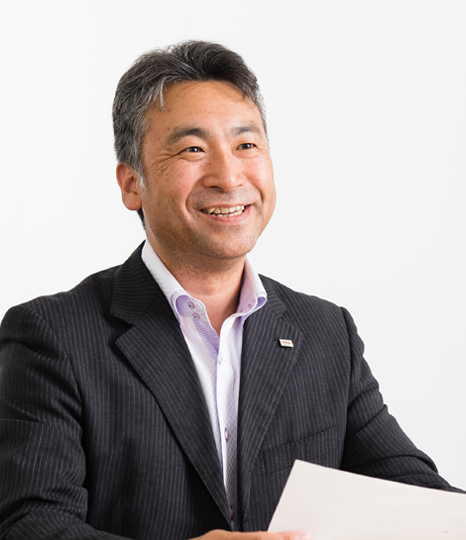
In 2004, when this deregulation started, the power company began joint development with Toshiba of a system for processing meter data received from smart meters. To calculate wheeling fees, the data processing platform used a relational database (RDB); using one month of the meter data which was sent every 30 minutes. Considering full retail liberalization, in April 2015 the company planned to have smart meters installed in all consumers in its region by 2023.
The number of smart meters jumped from several thousand to several millions and the amount of data to be processed increased several hundred-fold. In order to continue to process the data at high speeds, within specified time period, the company considered employing Toshiba's GridDB, a database management system to replace RDB. The power company and Toshiba understood that even if the RDB achieved its maximum performance, it would be still difficult to reach at the required performance level, tens of times greater than that of the existing system.
However, building a system with GridDB did not go smoothly.
At that time, smart community related demonstration trials and service launch projects were at its peak in Toshiba. GridDB was often used as a big data processing system for data obtained from various sensors, but reviews within the company were not all glowing. We received negative feedbacks as the system didn't perform as expected or as operational easiness didn't be given sufficient consideration. Why did the system face these problems, despite GridDB's exceptional processing capabilities? This question needed to be fixed before the power company deployed GridDB targeting full electricity deregulation. A hypothesis for the performance problems was developed: “The system is not optimal conditions for GridDB to perform its full capabilities.”
Click here to move to the top of this page.
Providing optimized integration using template systems
Looking back through our past verification projects, we had applied GridDB as an extension of existing systems and technologies. There were cases in which we built systems relying GridDB's exceptional capabilities however, without sufficiently consideration of its strengths and actual use cases. As a result, a conclusion we reached, we were unable to bring out the full performance of GridDB.
Based on this hypothesis, we carried out repeated rounds of verification and improvement, developing a template system through which GridDB's full performance could be leveraged for big data processing. After testing that we achieved reliable results, we created a strategy for applying this approach to the power company's wheeling system. These activities were led by four implementation policies: “Do not use GridDB for everything, but choose specific projects that is expected to produce exceptional results,” “Identify which fields RDB and GridDB excel in and consider total results by the appropriate database combination,” “Clarify the operation requirements of electrical power retail liberalization-related systems and make improvements to GridDB as necessary,” and “To accrue expertise and experience through the projects and deploy to other systems, set up and organize guidelines for Grid DB.”
The template system for the GridDB-based big data processing wheeling system used Toshiba's SmartEDA data collection and processing software. For application the system used Toshiba's GridData analysis solution with capability of parallel distributed processing of large amounts of data, and it works well with GridDB. This template system performs high speed processing of the large volumes of meter data sent by 7.5 million smart meters. Optimized integration was performed, using the appropriate materials for the appropriate situations, including existing technologies. For example, RDB was used for screen processing and data perpetuation. Furthermore, we carried out integration to meet operation requirements which clearly defined targets and achievement levels based on the Information-technology Promotion Agency (IPA) Non-Functional Requirements Grade*. We also verified the scalability of the system, which can be achieved through both scaling up (replacing hardware) and scaling out (adding hardware) (Fig. 2).
* The Non-Functional Requirements Grade is a collection of tools for non-functional requirement visualization and confirmation published by the Information-technology Promotion Agency (IPA) for the purposes of preventing development from being carried out while misunderstandings exist or differing interpretations of intent exist between contracting and contracted parties with regard to non-functional requirements requirements other than work function requirements when developing information systems.
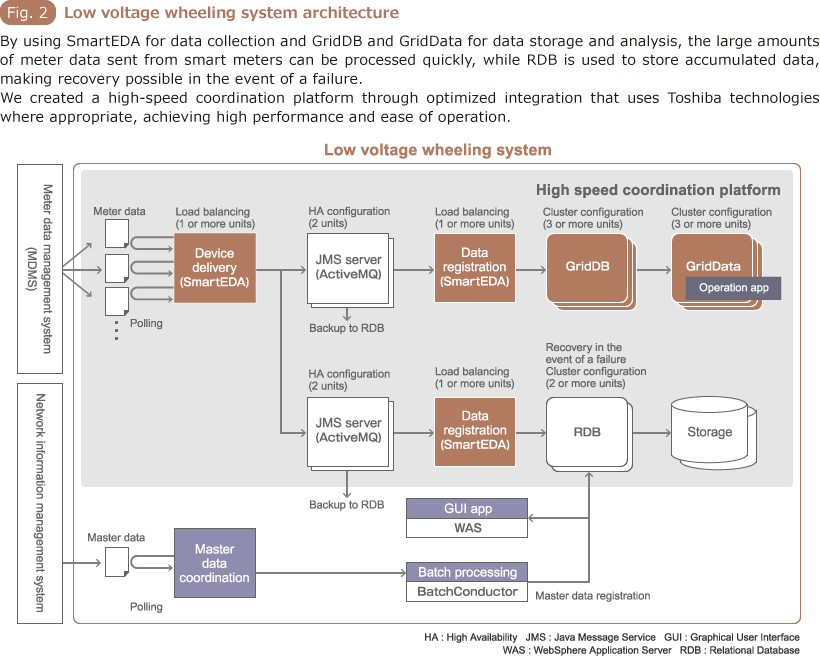
Click here to move to the top of this page.
Achieving overwhelming performance on on-site systems
One year after the start of these verification and improvement activities, performance evaluating testing was carried out. The target level of the test were to capture meter data from 7.5 million meters within 10 minutes and process them within 5 minutes. The system captured the data in 3 minutes and 44 seconds, and processed them in 1 minute and 32 seconds. GridDB's excellent processing performance producing stunning results, far beyond the target level, for the test wheeling system. Based on the results of this test the power company decided to apply the template system to an on-site system. This time, difference in behavior between the on-site peripheral systems and those planned in the use case caused shortfall of envisioned performance. This problem was rapidly resolved by redesigning containers to fit the actual use case. In April 2016 the system went into commercial operation. Over a three month period 13 billion records, totaling 2.6 terabytes, were collected via SmartEDA in the form of meter data sent every 30 minutes by several million smart meters, and stored in GridDB system. The wheeling system uses GridData's parallel distributed processing functions to calculate electricity usage amounts and costs and notify electrical power retailers in timely fashion. The system has been in operation stably for over a year, day and night, as it performs its heavy processing tasks.
GridDB is a database management system capable of ultra-high speed processing of large amounts of data. No matter how exceptional a technology may be however, to achieve performances as envisioned, it is the key to identify appropriate use case. Sufficient demonstration trial must be carried out in advance, integration must be applied by considering individual situations, and uncompromising effort and continuous improvement is required until the system reaches desired results. In this case, combination with GridData and RDB, GridDB was used only in a field in which its exceptional capabilities could be sufficiently exerted. As a result, the system produced excellent performance through overall optimization, not isolated optimization. Application design and database design were unified using a service oriented-approach, which we believe is the correct approach for leveraging GridDB.
The age of IoT is upon us. This electrical power system has demonstrated GridDB's capability of processing huge amounts of data even for cases with heavy responsibility. Performance demands is rising, making it necessary to meet even more demanding operation requirements. We will continue to do our utmost to use GridDB to accelerate the IoT-based digital transformation with our customers.
* GridDB is introduced in detail in #03.
* The corporate names, organization names, job titles and other names and titles appearing in this article are those as of August 2017.
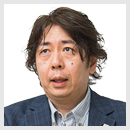
